Note
Go to the end to download the full example code.
Tutorial for UWB Data Preprocessing¶
import torch
import torch.nn as nn
import numpy as np
import os
import sys
import matplotlib.pyplot as plt
Plot function¶
def plot_uwb_heatmap(uwb_data):
"""
Plot UWB heatmap.
"""
data_shape = uwb_data.shape
if len(data_shape) == 2:
uwb_to_plot = uwb_data
elif len(data_shape) == 3:
uwb_to_plot = np.mean(uwb_data, axis=0)
else:
raise ValueError("The input data should have at least 2 dimensions.")
plt.figure(figsize=(15, 8))
plt.imshow(uwb_to_plot, aspect='auto', cmap='viridis')
plt.colorbar(label='UWB Amplitude')
plt.title('UWB Heatmap')
plt.xlabel('Time Index')
plt.ylabel('Channel Index')
plt.show()
Load UWB Data¶
root_dir = './data/sleep_pose_net_data'
data_dir = os.path.join(root_dir, 'Dataset I')
x = np.load(os.path.join(data_dir, 'X.npy'))
x = x[:,:,30:130]
x_amp_sample = np.abs(x)[1,:,:]
plot_uwb_heatmap(x_amp_sample)
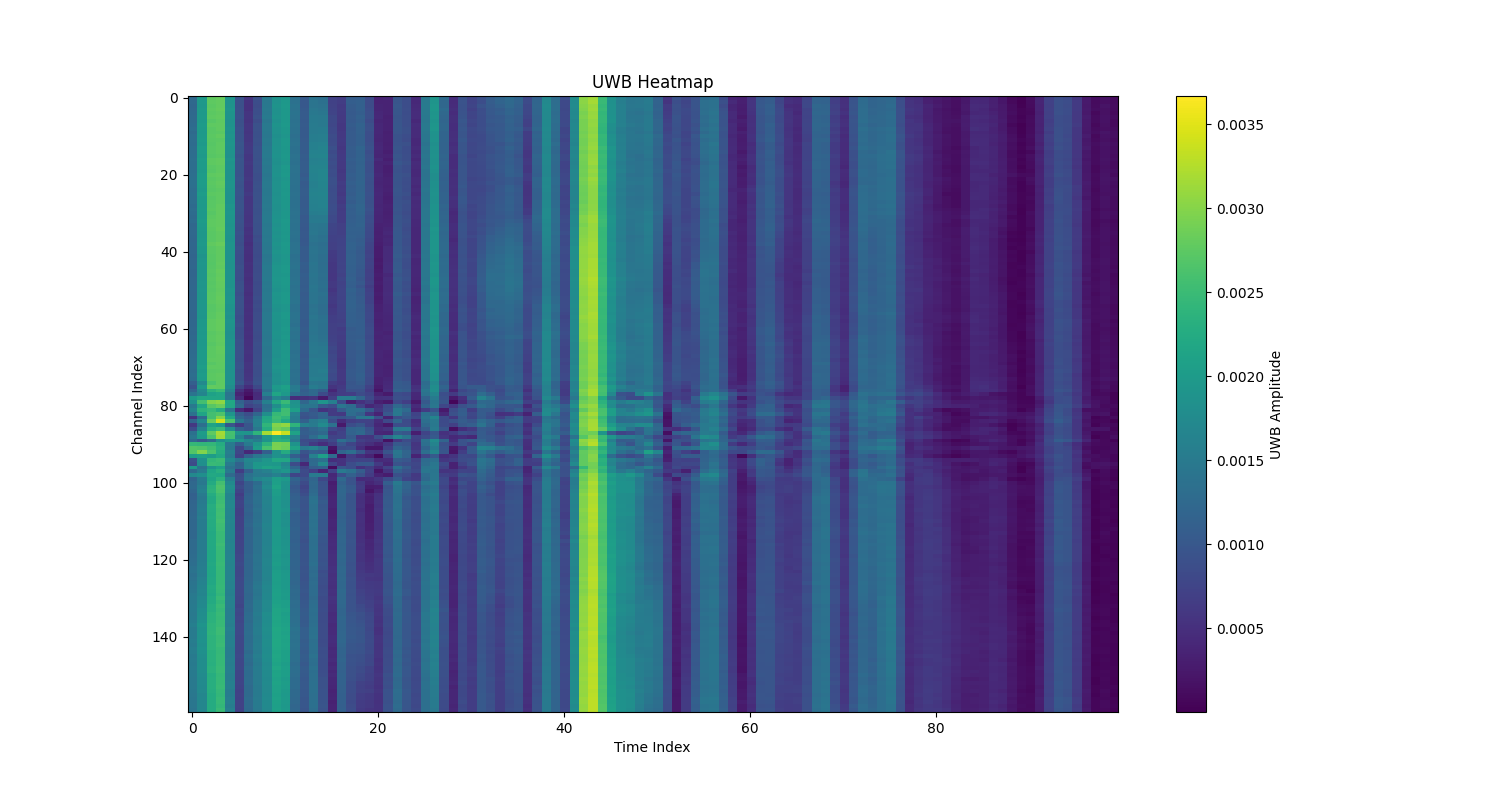
Clutter Suppression¶
Load corresponding Clutter Suppression functions
from pysensing.uwb.preprocessing.clutter_suppression import *
DC Suppression¶
x_amp_sample_ = np.expand_dims(x_amp_sample, axis=0)
dc_suppressed_x = dc_suppression(x_amp_sample_)
plot_uwb_heatmap(dc_suppressed_x)
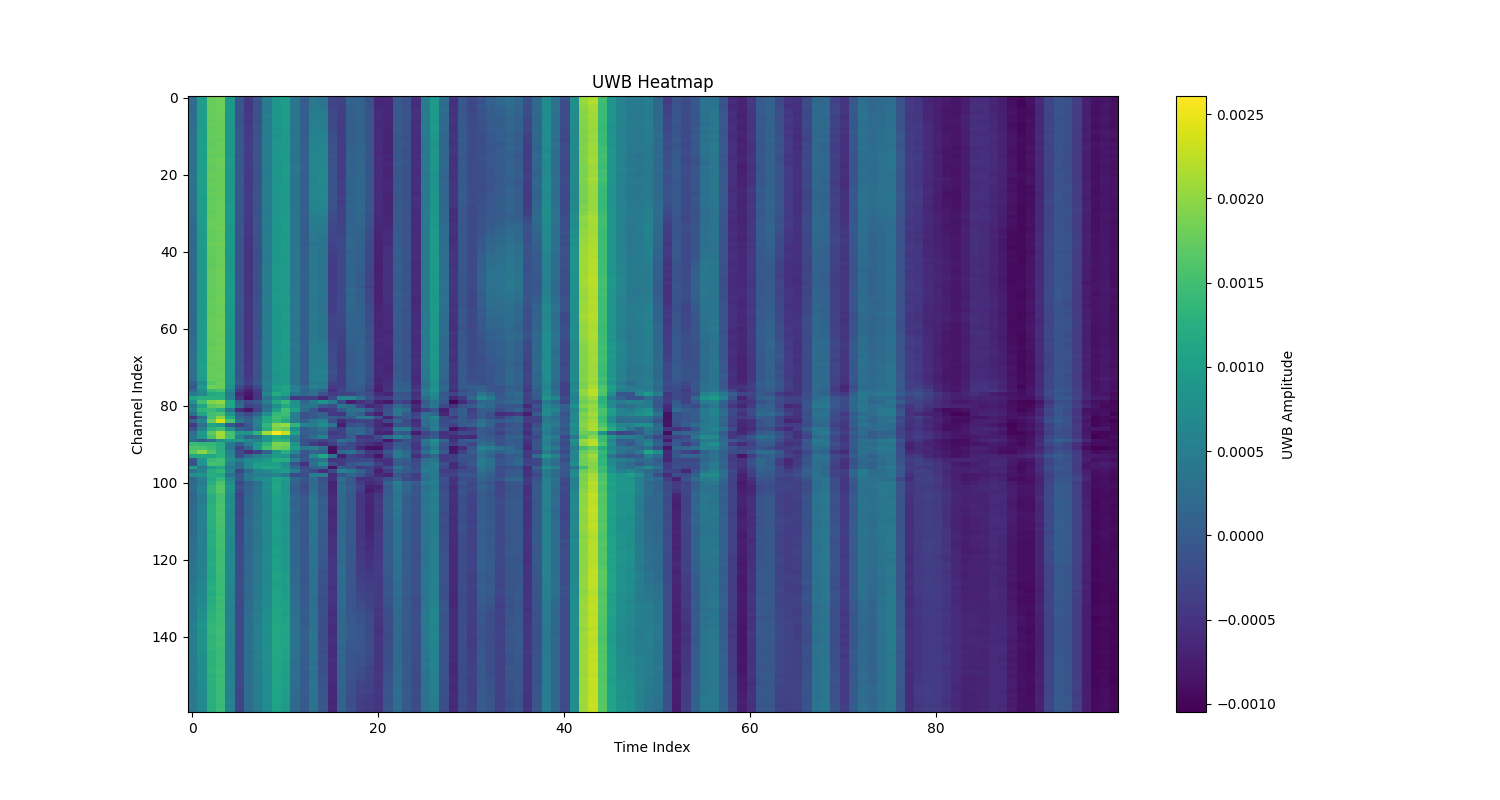
Static Background Suppression¶
x_amp_sample_copy = np.expand_dims(x_amp_sample, axis=0)
static_background_suppressed_x = static_background_suppression(x_amp_sample_copy)
plot_uwb_heatmap(static_background_suppressed_x)
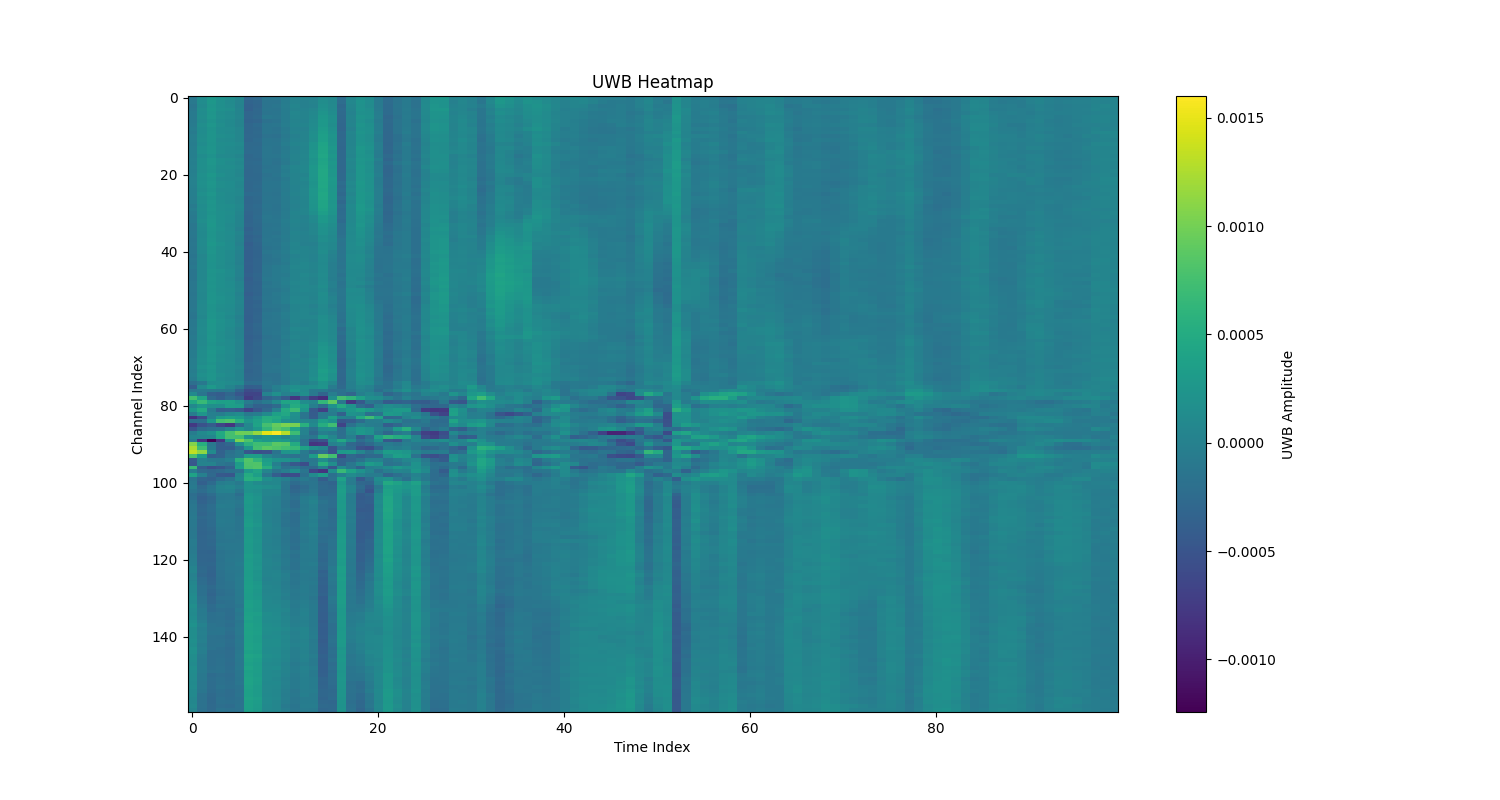
Running Background Suppression¶
x_amp_sample_copy = np.expand_dims(x_amp_sample, axis=0)
running_background_suppressed_x = running_background_suppression(x_amp_sample_copy, alpha=0.1)
plot_uwb_heatmap(running_background_suppressed_x)
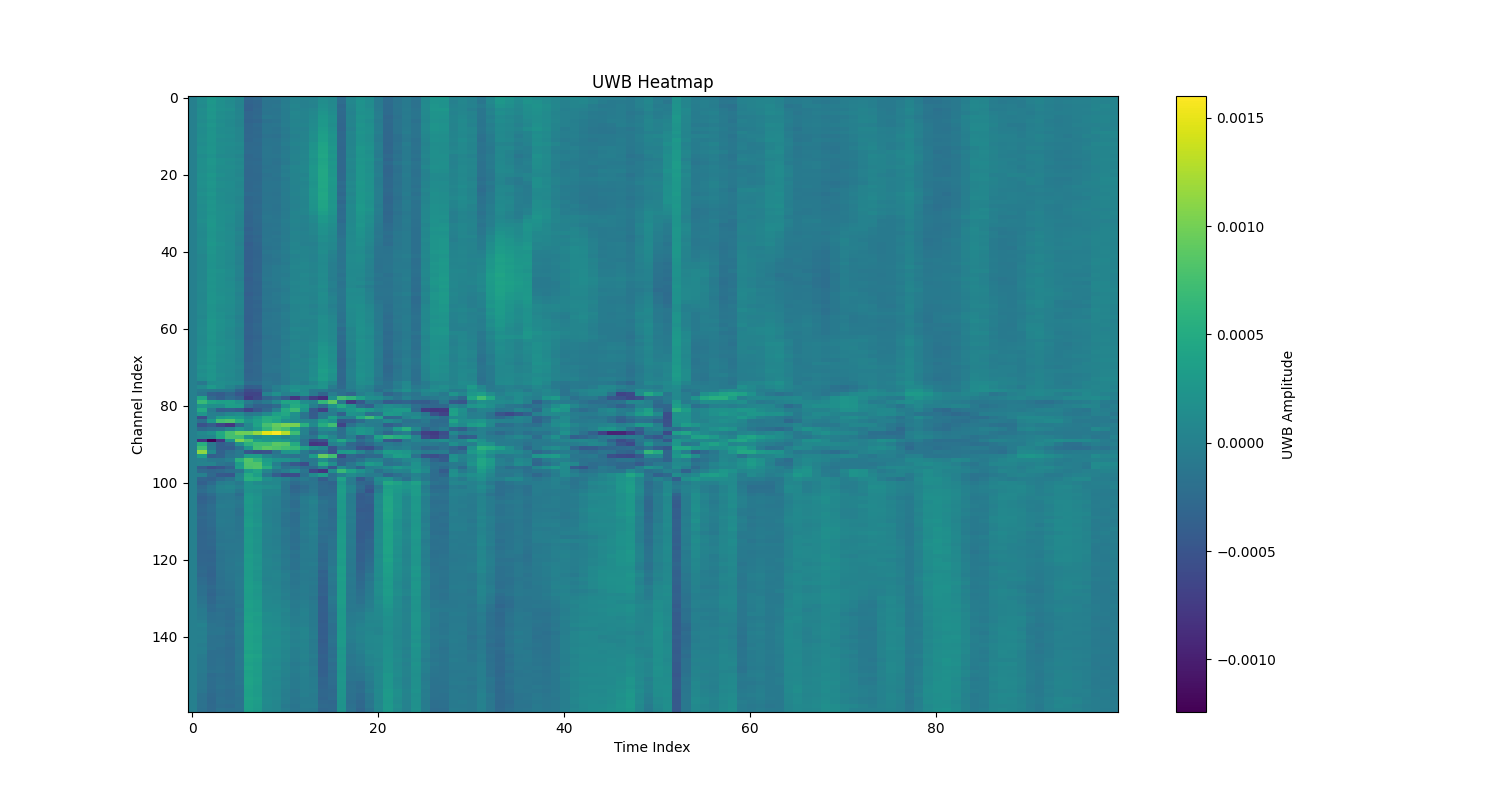
Cropping¶
Load corresponding Cropping functions
from pysensing.uwb.preprocessing.cropping import *
Range Selection¶
x_amp_sample_ = np.expand_dims(x_amp_sample, axis=0)
cropped_x, spatial_highest_position = range_selection(x_amp_sample_, spatial_size = 50)
plot_uwb_heatmap(cropped_x)
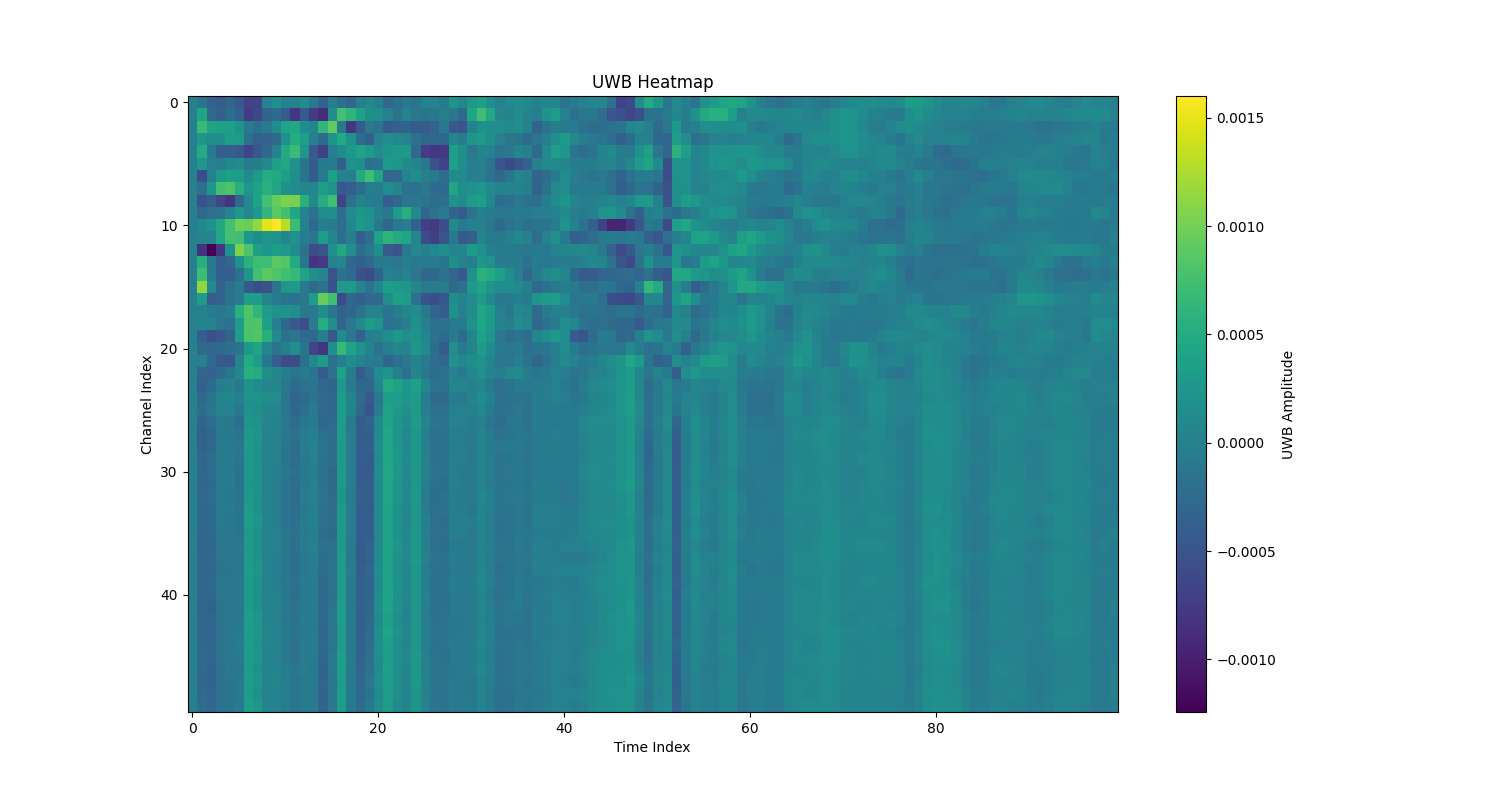
Filtering¶
Load corresponding Filtering functions
from pysensing.uwb.preprocessing.filtering import *
Band Pass Butterworth Filtering¶
x_amp_sample_ = np.expand_dims(x_amp_sample, axis=0)
butterworth_filter = bandpass_butterworth_filter(low_cut=2, high_cut=4, sample_rate=10, order=4)
filtered_uwb_data = butterworth_filter(x_amp_sample_)
plot_uwb_heatmap(filtered_uwb_data)
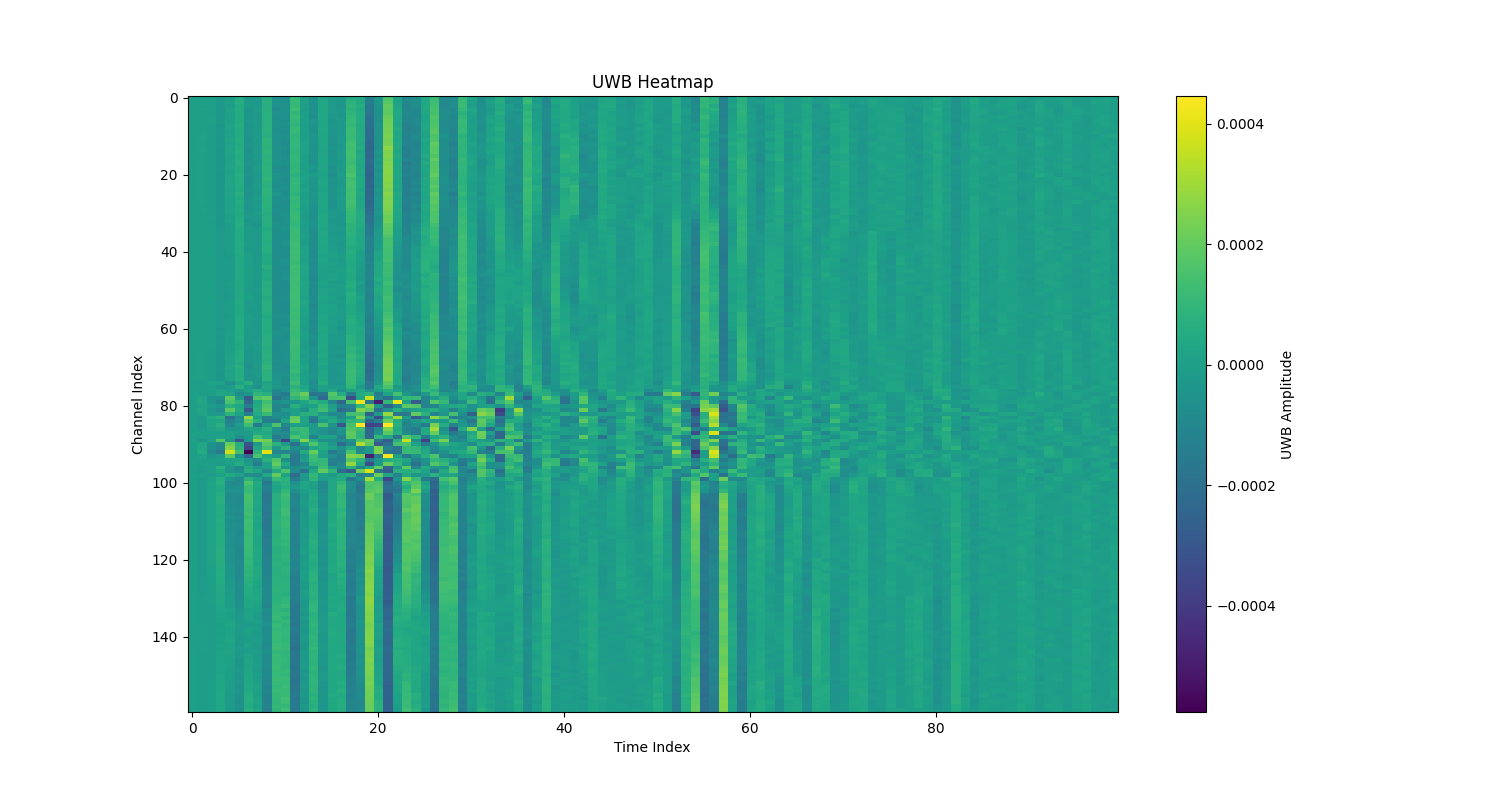
NaN Removal¶
from pysensing.uwb.preprocessing.nan_removal import *
x_amp_sample_ = x_amp_sample
nan_removed_data = np.zeros(x_amp_sample_.shape)
for i in range(len(x_amp_sample_)):
nan_removed_data[i,:] = remove_nan(x_amp_sample_[i,:])
plot_uwb_heatmap(nan_removed_data)
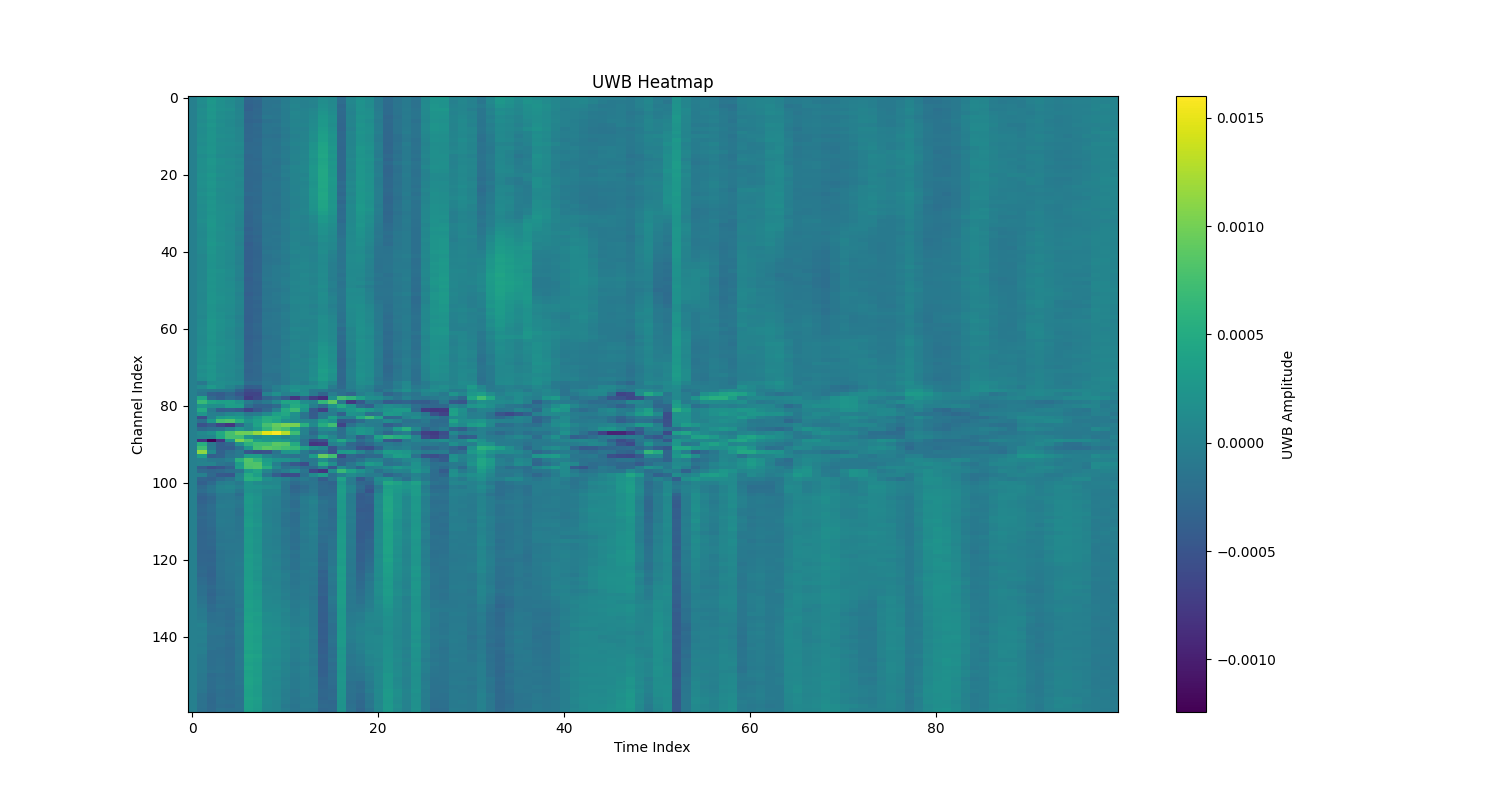
Normalization¶
from pysensing.uwb.preprocessing.normalization import *
x_amp_sample_ = x_amp_sample
normalized_uwb_data = normalize_data(x_amp_sample_)
plot_uwb_heatmap(normalized_uwb_data)
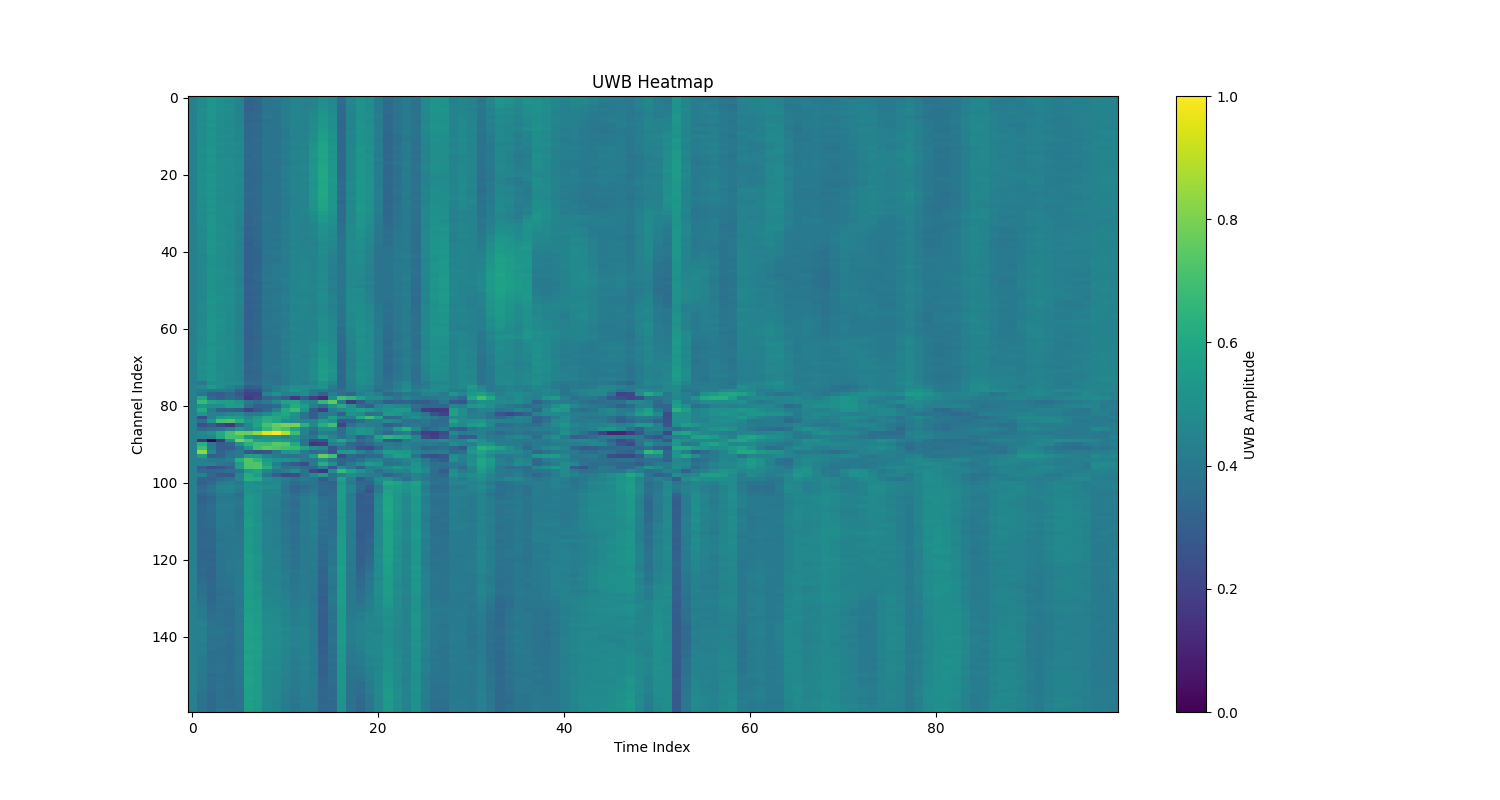
Transformation¶
Load corresponding Transformation functions
from pysensing.uwb.preprocessing.transformation import *
Time Difference Transform¶
x_input = np.abs(x)
time_difference_uwb_data = time_difference_transform(x_input, norm = True)
plot_uwb_heatmap(time_difference_uwb_data[0])
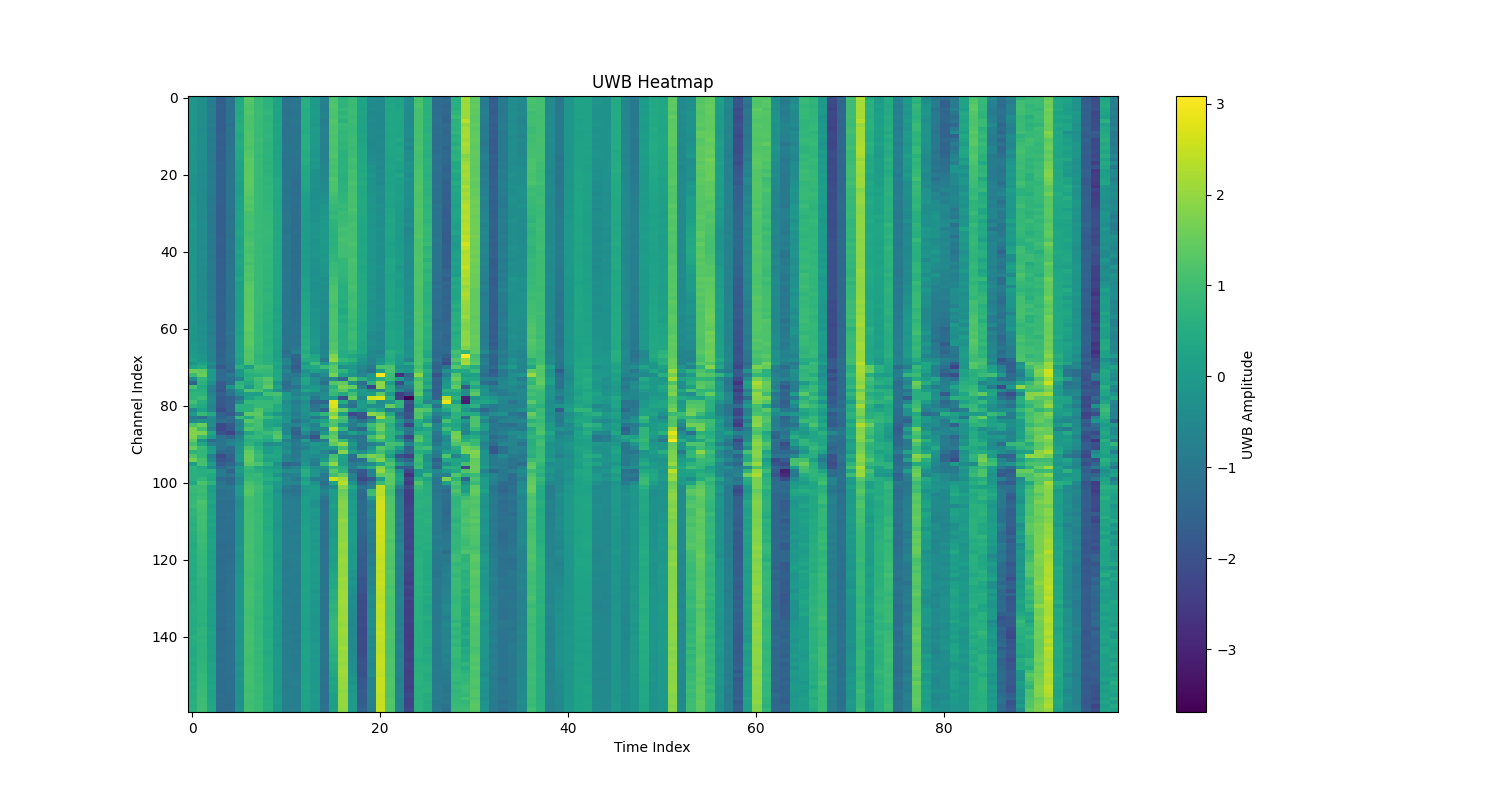
Weighted RTF Transform¶
x_input = np.abs(x)
wrtft_uwb_data = weighted_rtf_transform(x_input, NFFT = 25, stride = 2, norm = True)
plot_uwb_heatmap(wrtft_uwb_data[0])
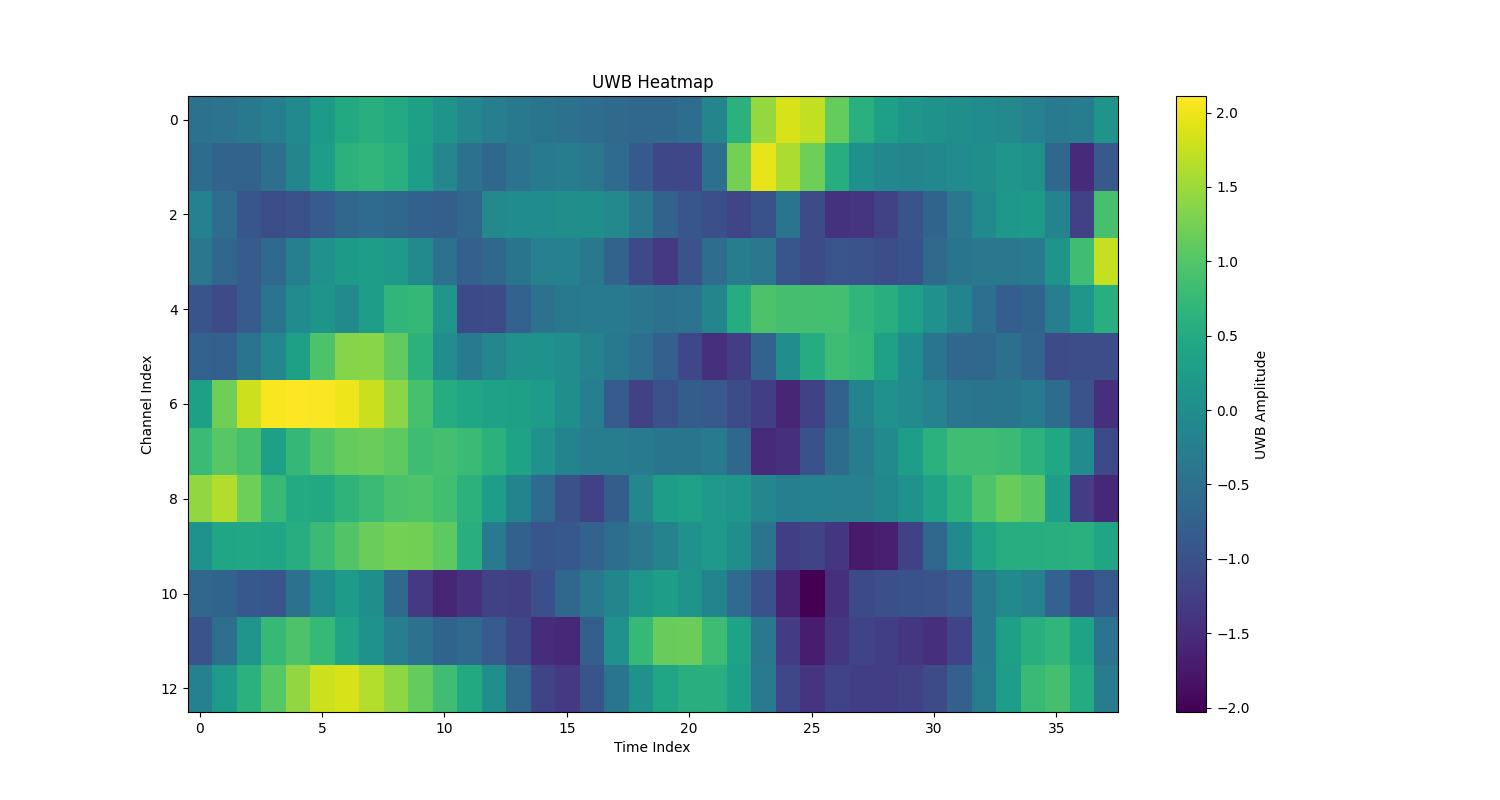
0%| | 0/930 [00:00<?, ?it/s]
1%| | 8/930 [00:00<00:12, 73.01it/s]
2%|▏ | 16/930 [00:00<00:12, 73.53it/s]
3%|▎ | 24/930 [00:00<00:12, 72.88it/s]
3%|▎ | 32/930 [00:00<00:12, 72.92it/s]
4%|▍ | 40/930 [00:00<00:12, 73.37it/s]
5%|▌ | 48/930 [00:00<00:11, 73.57it/s]
6%|▌ | 56/930 [00:00<00:11, 73.48it/s]
7%|▋ | 64/930 [00:00<00:11, 73.57it/s]
8%|▊ | 72/930 [00:00<00:11, 73.57it/s]
9%|▊ | 80/930 [00:01<00:11, 73.49it/s]
9%|▉ | 88/930 [00:01<00:11, 73.58it/s]
10%|█ | 96/930 [00:01<00:11, 73.62it/s]
11%|█ | 104/930 [00:01<00:11, 73.65it/s]
12%|█▏ | 112/930 [00:01<00:11, 73.52it/s]
13%|█▎ | 120/930 [00:01<00:11, 73.54it/s]
14%|█▍ | 128/930 [00:01<00:10, 74.00it/s]
15%|█▍ | 136/930 [00:01<00:10, 74.65it/s]
15%|█▌ | 144/930 [00:01<00:10, 74.82it/s]
16%|█▋ | 152/930 [00:02<00:10, 74.79it/s]
17%|█▋ | 160/930 [00:02<00:10, 74.76it/s]
18%|█▊ | 168/930 [00:02<00:10, 74.71it/s]
19%|█▉ | 176/930 [00:02<00:10, 74.65it/s]
20%|█▉ | 184/930 [00:02<00:10, 74.56it/s]
21%|██ | 192/930 [00:02<00:09, 74.64it/s]
22%|██▏ | 200/930 [00:02<00:09, 74.51it/s]
22%|██▏ | 208/930 [00:02<00:09, 74.67it/s]
23%|██▎ | 216/930 [00:02<00:09, 74.70it/s]
24%|██▍ | 224/930 [00:03<00:09, 74.63it/s]
25%|██▍ | 232/930 [00:03<00:09, 74.65it/s]
26%|██▌ | 240/930 [00:03<00:09, 74.88it/s]
27%|██▋ | 248/930 [00:03<00:09, 75.26it/s]
28%|██▊ | 256/930 [00:03<00:08, 75.83it/s]
28%|██▊ | 264/930 [00:03<00:08, 75.97it/s]
29%|██▉ | 272/930 [00:03<00:08, 76.25it/s]
30%|███ | 280/930 [00:03<00:08, 76.92it/s]
31%|███ | 288/930 [00:03<00:08, 77.55it/s]
32%|███▏ | 296/930 [00:03<00:08, 77.86it/s]
33%|███▎ | 304/930 [00:04<00:08, 77.63it/s]
34%|███▎ | 312/930 [00:04<00:07, 77.26it/s]
34%|███▍ | 320/930 [00:04<00:07, 77.17it/s]
35%|███▌ | 328/930 [00:04<00:07, 76.53it/s]
36%|███▌ | 336/930 [00:04<00:07, 76.72it/s]
37%|███▋ | 344/930 [00:04<00:07, 76.58it/s]
38%|███▊ | 352/930 [00:04<00:07, 76.41it/s]
39%|███▊ | 360/930 [00:04<00:07, 76.64it/s]
40%|███▉ | 368/930 [00:04<00:07, 76.92it/s]
40%|████ | 376/930 [00:05<00:07, 76.71it/s]
41%|████▏ | 384/930 [00:05<00:07, 76.45it/s]
42%|████▏ | 392/930 [00:05<00:07, 76.44it/s]
43%|████▎ | 400/930 [00:05<00:06, 76.55it/s]
44%|████▍ | 408/930 [00:05<00:06, 76.80it/s]
45%|████▍ | 416/930 [00:05<00:06, 77.02it/s]
46%|████▌ | 424/930 [00:05<00:06, 77.11it/s]
46%|████▋ | 432/930 [00:05<00:06, 77.21it/s]
47%|████▋ | 440/930 [00:05<00:06, 77.19it/s]
48%|████▊ | 448/930 [00:05<00:06, 77.18it/s]
49%|████▉ | 456/930 [00:06<00:06, 77.21it/s]
50%|████▉ | 464/930 [00:06<00:06, 76.98it/s]
51%|█████ | 472/930 [00:06<00:05, 76.74it/s]
52%|█████▏ | 480/930 [00:06<00:05, 76.86it/s]
52%|█████▏ | 488/930 [00:06<00:05, 76.96it/s]
53%|█████▎ | 496/930 [00:06<00:05, 76.90it/s]
54%|█████▍ | 504/930 [00:06<00:05, 76.77it/s]
55%|█████▌ | 512/930 [00:06<00:05, 76.67it/s]
56%|█████▌ | 520/930 [00:06<00:05, 76.65it/s]
57%|█████▋ | 528/930 [00:06<00:05, 76.69it/s]
58%|█████▊ | 536/930 [00:07<00:05, 76.90it/s]
58%|█████▊ | 544/930 [00:07<00:05, 76.51it/s]
59%|█████▉ | 552/930 [00:07<00:04, 76.09it/s]
60%|██████ | 560/930 [00:07<00:04, 75.74it/s]
61%|██████ | 568/930 [00:07<00:04, 75.32it/s]
62%|██████▏ | 576/930 [00:07<00:04, 75.05it/s]
63%|██████▎ | 584/930 [00:07<00:04, 74.68it/s]
64%|██████▎ | 592/930 [00:07<00:04, 74.61it/s]
65%|██████▍ | 600/930 [00:07<00:04, 74.77it/s]
65%|██████▌ | 608/930 [00:08<00:04, 74.83it/s]
66%|██████▌ | 616/930 [00:08<00:04, 74.82it/s]
67%|██████▋ | 624/930 [00:08<00:04, 75.02it/s]
68%|██████▊ | 632/930 [00:08<00:03, 75.08it/s]
69%|██████▉ | 640/930 [00:08<00:03, 75.02it/s]
70%|██████▉ | 648/930 [00:08<00:03, 75.02it/s]
71%|███████ | 656/930 [00:08<00:03, 74.56it/s]
71%|███████▏ | 664/930 [00:08<00:03, 74.52it/s]
72%|███████▏ | 672/930 [00:08<00:03, 74.88it/s]
73%|███████▎ | 680/930 [00:09<00:03, 75.25it/s]
74%|███████▍ | 688/930 [00:09<00:03, 75.77it/s]
75%|███████▍ | 696/930 [00:09<00:03, 76.18it/s]
76%|███████▌ | 704/930 [00:09<00:02, 76.15it/s]
77%|███████▋ | 712/930 [00:09<00:02, 76.22it/s]
77%|███████▋ | 720/930 [00:09<00:02, 75.95it/s]
78%|███████▊ | 728/930 [00:09<00:02, 76.04it/s]
79%|███████▉ | 736/930 [00:09<00:02, 76.22it/s]
80%|████████ | 744/930 [00:09<00:02, 76.06it/s]
81%|████████ | 752/930 [00:09<00:02, 76.30it/s]
82%|████████▏ | 760/930 [00:10<00:02, 76.03it/s]
83%|████████▎ | 768/930 [00:10<00:02, 75.88it/s]
83%|████████▎ | 776/930 [00:10<00:02, 75.78it/s]
84%|████████▍ | 784/930 [00:10<00:01, 75.68it/s]
85%|████████▌ | 792/930 [00:10<00:01, 75.59it/s]
86%|████████▌ | 800/930 [00:10<00:01, 75.49it/s]
87%|████████▋ | 808/930 [00:10<00:01, 75.60it/s]
88%|████████▊ | 816/930 [00:10<00:01, 75.69it/s]
89%|████████▊ | 824/930 [00:10<00:01, 75.26it/s]
89%|████████▉ | 832/930 [00:11<00:01, 74.98it/s]
90%|█████████ | 840/930 [00:11<00:01, 75.06it/s]
91%|█████████ | 848/930 [00:11<00:01, 75.80it/s]
92%|█████████▏| 856/930 [00:11<00:00, 76.28it/s]
93%|█████████▎| 864/930 [00:11<00:00, 76.18it/s]
94%|█████████▍| 872/930 [00:11<00:00, 76.28it/s]
95%|█████████▍| 880/930 [00:11<00:00, 76.57it/s]
95%|█████████▌| 888/930 [00:11<00:00, 76.72it/s]
96%|█████████▋| 896/930 [00:11<00:00, 76.91it/s]
97%|█████████▋| 904/930 [00:11<00:00, 76.51it/s]
98%|█████████▊| 912/930 [00:12<00:00, 76.64it/s]
99%|█████████▉| 920/930 [00:12<00:00, 76.57it/s]
100%|█████████▉| 928/930 [00:12<00:00, 76.55it/s]
100%|██████████| 930/930 [00:12<00:00, 75.66it/s]
Total running time of the script: (0 minutes 13.547 seconds)